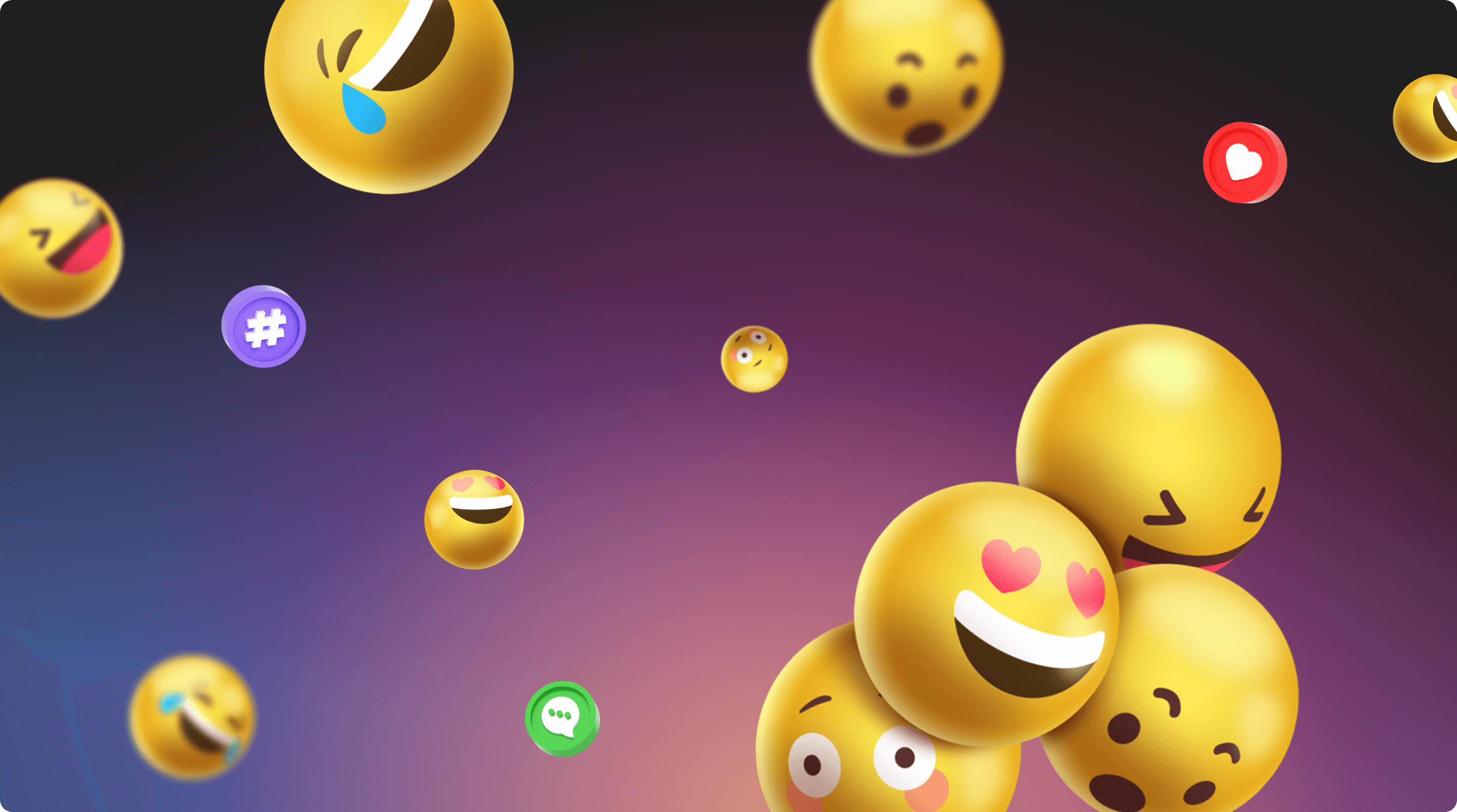
What Is Sentiment Analysis?
When it comes to promoting the brand, reputation management, and further development strategy, any company faces the challenge of the abundance of data available on the internet.
There is overwhelming information, from social media posts to customer reviews. It is challenging to make sense of all this data, especially when understanding people’s opinions and emotions. Sentiment analysis helps to cope with the task.
Sentiment analysis uses natural language processing (NLP) and machine learning techniques to analyse and understand people’s sentiments, opinions, and emotions expressed in text. It is also known as opinion mining or emotion AI.
The main goal of sentiment analysis is to determine the emotional tone behind a text. It could be anything from a tweet about a brand to a product review on Amazon. The sentiment analysis process breaks down the text into individual words and phrases, then applies algorithms to analyse the context and identify the sentiment behind each one.
There are several approaches to sentiment analysis, including rule-based systems, machine learning, and deep learning techniques. Let’s take a closer look at each of these approaches:
- Rule-based systems
Rule-based systems use pre-defined rules to identify positive, negative, or neutral sentiments based on words and phrases commonly associated with each of them.
For example, a rule-based system may identify the word “love” as positive and the word “hate” as negative. Experts typically create these rules in the field or through statistical analysis of large datasets.
- Machine learning
Machine learning involves training a model on a large dataset of labelled data to identify sentiment based on patterns and trends in the data.
These models are typically trained on datasets containing millions of text examples, with each example labelled as positive, negative, or neutral. The model then uses these examples to learn patterns and trends that can be used to identify sentiment in new text examples.
- Deep learning
Deep learning is a subset of machine learning that uses artificial neural networks to identify sentiment by analysing the context and meaning of words and phrases.
These models are typically trained on large datasets and can identify more complex patterns and relationships between words and phrases. Deep learning models have been shown to outperform other approaches to sentiment analysis in some cases.
- Each approach to sentiment analysis has its strengths and weaknesses. Rule-based systems are often easy to implement and interpret, but they can be limited by the quality of the rules and the complexity of the language being analysed.
Machine learning and deep learning approaches can be more powerful, but they require large amounts of labelled data and can be more challenging to interpret.
The choice of approach will depend on the specific use case and the resources available. In many cases, combining these approaches may be used to achieve the best results. Sentiment analysis is used in various applications, from marketing and advertising to customer service and product development.
For example, businesses can use sentiment analysis to monitor customer sentiment toward their products or services on social media and other online platforms. This information can then be used to improve products, respond to customer complaints, and identify trends and patterns in customer behaviour.
The approach is also used in politics to gauge public opinion on political issues and candidates. It can also be applied in healthcare to analyse patient feedback and identify areas for improvement in the healthcare system.
Sentiment analysis is a powerful tool enabling businesses and organisations to understand their customers and stakeholders better. Companies can improve their products, services, and communication strategies by analysing people’s sentiments, opinions, and emotions expressed in text.